According to a McKinsey report [1], construction is one of the biggest industries in the world, being responsible for 13% of the global Gross Domestic Product, and yet, even when outside of crises, it does not perform well. Improving the effectiveness of production control has attracted the interest of researchers and lean construction practitioners over the years. In lean construction, production activities are improved continuously with respect to waste and value [2].
With the advent of Industry 4.0, companies have been channeling their efforts to achieve superior performance by advancing levels of automation and interconnectivity. With the incorporation of Industry 4.0 technologies, process stability increases and potential issues that jeopardize delivering according to customers’ needs can be anticipated [3]. The use of digital technologies to measure waste on worker, subcontractor and project level could provide significant benefits to an industry plagued with poor productivity [4].
This post gives an overview of the main existing methods in the literature for measuring, analyzing, and improving productivity on construction sites using digital technologies for automated data collection.
PRODUCTIVITY MONITORING IN CONSTRUCTION
Definitions of productivity range from industry-wide economic parameters to the measurement of crews and individuals, and each of these measures has its unique purpose. At the project site, contractors are often interested in labor productivity, which can be expressed as the ratio between outputs expressed in specific physical units and inputs expressed in man-hours [5].
Work sampling, as a technique used to indirectly assess productivity, consists of observing the activities at regular intervals and categorizing them into different work categories to evaluate how time is utilized [6]. Each observation records what is happening at that instant, and the technique is based upon statistical sampling theory [5]. Compared to work sampling, the activity analysis technique includes more detailed observations, provides a more descriptive assessment of the effectiveness of the utilization of workers' time, and can continuously identify the areas for productivity improvements [7].
Regarding the calculation of productivity rates for machinery performing cyclic activities, it is first necessary to estimate the cycle times [8]. On earthmoving activities, the soil amount, which can be estimated based on the number of dump trucks loading and their soil-capacity, and the operating hours are two main aspects that must be considered for productivity monitoring [9].
As it can be noted, traditional methods for productivity assessment at construction sites, despite being effective, are time-consuming and based on manual data collection and direct observation of activities on-site, which hampers the obtaining of reliable and up-to-date information of activities productivity.
METHODS USING COMPUTER VISION-BASED TECHNOLOGIES
Methods using computer vision-based technologies classify the actions of workers and equipment on videos into categories that may be used for activity analysis. These methods require algorithms for detecting and tracking resources, and procedures for activity recognition.
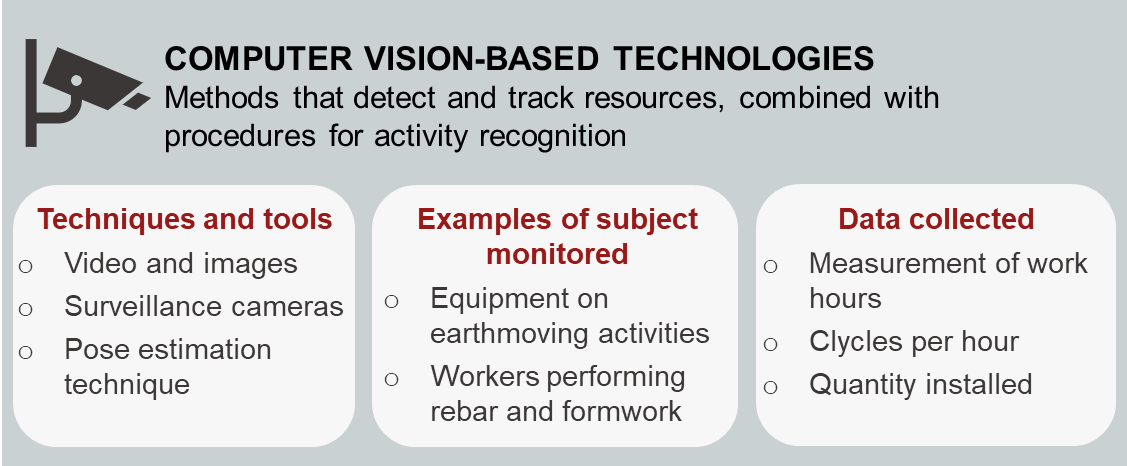
Figure 1 – Computer vision-based technologies for productivity monitoring
In this approach it is possible to perform automated measurement of inputs and outputs to calculate the productivity of activities using algorithms for measuring installed work quantity and working hours of construction workers. The productivity data can be linked with the 4D BIM model, which helps to predict construction scheduling for management purposes. Methods can be developed for estimating the productivity of soil removal by combining photogrammetry to measure the volume of the excavated soil, and video analysis to generate statistics regarding the construction activities.
Pose estimation techniques, commonly used in research on construction worker ergonomics, have also gained prominence among productivity studies. In these studies, authors use visual data collected by high resolution cameras to detect and track workers’ skeleton features to interpret and analyze their activities. Surveillance cameras may not provide detailed information for pose estimation methods, but can reduce costs with the use of cameras that already exist on construction sites.
One of the advantages of vision-based methods is that videos are understandable by any visually able person, provide detailed information, and allow reviews by managers away from the work sites. Visual data contains information about not only the physical movements of workers and equipment, but also their visual features and spatial-contextual natures. On the other hand, computer vision algorithms are sensitive to environmental factors such as occlusions, lighting, and illumination conditions. Shaking of cameras caused by wind, and blur of images caused by rain, snow, and fog represent additional challenges for equipment and worker action recognition. Besides that, a single camera can only cover a limited field of view. To fully cover a large construction job site, it would be necessary to install multiple cameras in various locations.
METHODS USING SENSOR-BASED TECHNOLOGIES
The use of body-worn sensors such as accelerometer, gyroscope, and magnetometer that enable the measurement of workers’ posture and motions has gained greater attention for construction activity monitoring. Accelerometers are resilient and robust in difficult conditions compared with image sensors, besides having a small size, good accuracy, and reasonable power consumption. Another advantage is that they can be embedded in wristbands to classify activities performed with hands, such as masonry, ironwork, and carpentry.
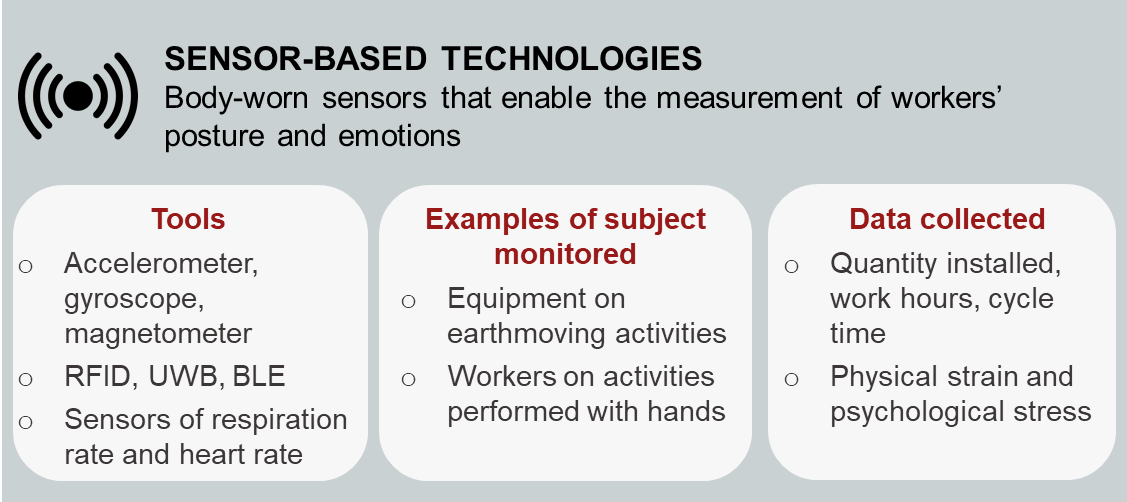
Figure 2 – Sensor-based technologies for productivity monitoring
Real-Time Location Sensors (RTLS) such as Radio Frequency Identification (RFID) and Ultra-Wideband (UWB) draw attention from researchers and practitioners because of their technological maturity, cost-efficient infrastructure, and ability to operate without line of sight. These sensors have been used to analyze the time trajectories of workers and to perform automated work sampling. Using RTLS it is possible to analyze the share of uninterrupted presence of workers in work locations, which is a necessary condition for value-added time, although not all time the workers spend in work locations is necessarily value-adding.
Studies have also been developed using biosensors in wearable devices to analyze factors that affect the productivity of construction workers. Heart rate is one of the physiological signals most used to study the influence of physical strain on productivity. Other physiological signals such as blood volume pulse, respiration rate, galvanic skin response, and skin temperature are also being tested to assess the effect of the emotional status of workers on their productivity level. Despite being promising, the use of too many sensors may be uncomfortable for the subject and can interfere with normal or spontaneous activity.
METHODS USING AUDIO-BASED TECHNOLOGIES
Audio has been investigated by researchers as input data for recognizing activities of construction heavy equipment that generate distinct acoustic patterns while performing routine tasks. Sounds generated by construction equipment can be recorded by commercially available microphones, allowing the classification of equipment operations into productive or major activities and non-productive or minor activities. Another possibility is the use of audio-based systems for estimating cycle times of construction equipment during its operation.
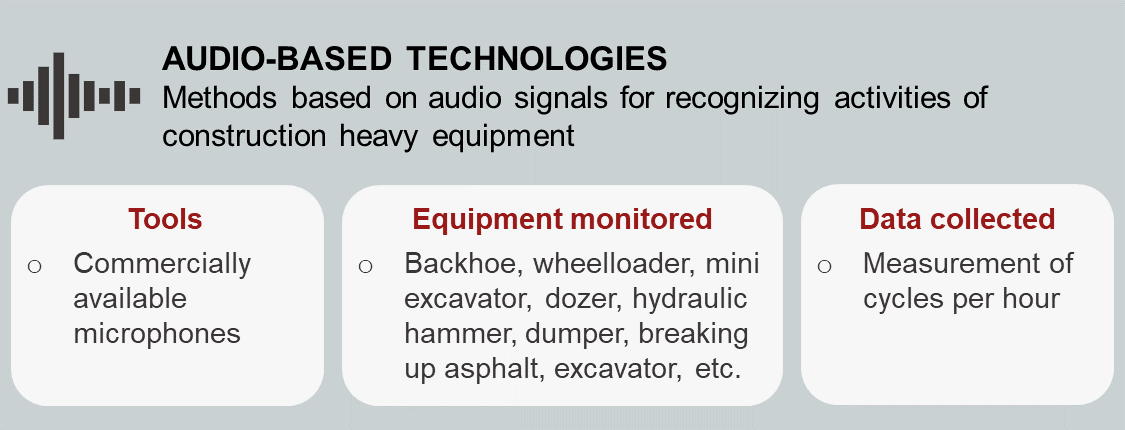
Figure 3 – Audio-based technologies for productivity monitoring
The application of audio signal processing techniques in the construction management area is still in the early stages of development. Compared to visual and kinematic data, sound provides certain advantages: a single microphone can cover larger areas without the need to be directly attached to a machine, and the processing of audio files is computationally less expensive compared to processing images and video files. However, the existence of background noise might be a negative factor for the algorithms, and certain types of construction machinery do not generate distinct sound patterns during operation.
DISCUSSION AND CONCLUSIONS
Results show that technologies based on computer vision and sensors are the most used for productivity monitoring on construction sites. These technologies can automate data collection for the processes of work sampling and activity analysis, as well as to measure inputs and outputs, and monitor physical and emotional factors that can influence workers’ productivity. Audio has been used for monitoring equipment productivity, especially for measuring cycle times. However, there are still few studies in this category.
Computer vision algorithms have made great advances in recent years, mainly with the use of deep learning techniques. Despite this fact, the detection of fine movements is still a challenge for vision-based methods. Pose estimation techniques, widely used in ergonomics studies, are capable of analyzing movements in a more detailed way. Due to their origin, pose estimation techniques have a great potential for studies of productivity monitoring integrated with ergonomics analysis. Regarding the use of sensors, further studies are needed to overcome the challenge of relating the worker's location to the type of work being performed, which could be done through the integration of RTLS with kinematic sensors. Studies using physiological signals have great potential to demonstrate the influence of stress and physical demand on workers' productivity.
Figure 4 – Workflow for integration of productivity and safety monitoring using digital technologies
Thus, as can be seen in Figure 4, there is an opportunity to combine the technologies of computer vision-based and sensor-based methods to provide evidence regarding the integrated management of productivity and safety and their impacts on the production process. This integration, despite being of great value, has been little explored in the literature.
References
[1] Ribeirinho, M. J., J. Mischke, G. Strube, E. Sjödin, J. L. Blanco, R. Palter, J. Biörck, D. Rockhill and T. Andersson (2020). The next normal in construction McKinsey & Company.
[2] Koskela, L. (1992). Application of the New Production Philosophy to Construction. Finland.
[3] Tortorella, G. L., R. Giglio and D. H. Van Dun (2019). "Industry 4.0 adoption as a moderator of the impact of lean production practices on operational performance improvement." Int. J. Oper. Prod. Manag. 39.
[4] Zhao, J., O. Seppänen, A. Peltokorpi, B. Badihi and H. Olivieri (2019). "Real-time resource tracking for analyzing value-adding time in construction." Autom. Constr. 104: 52-65.
[5] Thomas, H. R., W. F. Maloney, R. M. W. Horner, G. R. Smith, V. K. Handa and S. R. Sanders (1990). "Modeling construction labor productivity." J. Constr. Eng. Manage. 116: 705-726.
[6] Liou, F.-S. and J. P. Borcherdin (1986). "Work sampling can predict unit rate productivity." J. Constr. Eng. Manage. 112: 90-103.
[7] Cheng, T., J. Teizer, G. C. Migliaccio and U. C. Gatti (2013). "Automated task-level activity analysis through fusion of real time location sensors and worker's thoracic posture data" Autom. Constr. 29: 24–39.
[8] Sabillon, C., A. Rashidi, B. Samanta, M. A. Davenport and D. V. Anderson (2020). "Audio-Based Bayesian Model for Productivity Estimation of Cyclic Construction Activities " J. Comput. Civ. Eng. 34.
[9] Kim, J. and S. Chi (2020). "Multi-camera vision-based productivity monitoring of earthmoving operations" Autom. Constr. 112.